Updated in September 2024
Those days are long gone when artificial intelligence and machine learning sounded like sci-fi movies to us. Today we are going through the Fourth Industrial Revolution or simply Manufacturing 4.0, whose key drivers are large volumes of data and rapid technological advances. This couldn’t be more appropriate for the manufacturing sector, which has gotten closer to its timeless goal of producing more quality goods at lower expenses thanks to ML. So, what is this hype around machine learning and manufacturing, and how can your company unlock unprecedented growth if it introduces ML to its operations? Let’s look at a few machine learning use cases in manufacturing.
Top challenges in manufacturing
Aside from the massive shortages in skilled labor, the manufacturing industry in 2023 is experiencing a range of other challenges:
- Supply chain disruptions as a direct result of the COVID-19 pandemic
- Sustainability since more public and private sectors started to consider the environmental effect of their partner’s products and operations
- Inflation that appeared as a consequence of a limited supply chain in combination with the increased demand for products
Fortunately, machine learning can help you bridge these gaps at least partially, if not entirely. For example, technology can optimize production so machines will complete more complex tasks when it comes to the skill gap. By predicting production, a manufacturer also gets a chance to reduce waste for more sustainable manufacturing.
In other words, machine learning can help a company minimize unpredictability on the factory floor. This technology allows you to build more agile manufacturing processes and enable multi-target tracking, for example, as in the case of IoT supply chain management. Moreover, there are lots of other ways to apply machine learning in business, which we discuss below.
Use cases of ML in manufacturing
As a game-changing technology, machine learning brings next-gen optimization in manufacturing and, thus, has different use cases. Let’s consider the most critical examples of machine learning in the manufacturing process:
1. Predictive maintenance (PdM)
One of the AI/ML use cases in manufacturing is predictive maintenance. By using ML algorithms, a company checks the condition of equipment and predicts its future failure. This way, your staff can respond in advance, preventing downtime and extending equipment life.
Unlike reactive maintenance, predictive maintenance doesn’t expect to wait until the equipment deteriorates but monitors the equipment state to intervene timely. This means:
- Lower unplanned downtime and delayed production, usually caused by waiting until the new parts are bought and shipped for replacement or repairment
- Reduced maintenance costs since a manufacturer acts before the actual breakdown happens and interferes before the defect is too critical, i.e., more costly to repair
- Asset life extension since the degrading equipment parts get a timely replacement
- Fewer work injuries as a manufacturer has all the actual information about possible defects
Let’s compare predictive maintenance in manufacturing to preventive maintenance too. Here we talk about lower expenses since a manufacturer performs maintenance activities when they’re actually needed. Aside from avoiding unplanned downtime, PdM reduces downtime in general by minimizing scheduled maintenance activities, as in preventive maintenance.
An illustrative example is when Intelliarts helped an appliance manufacturer to implement predictive maintenance into their production. As a result, the company was able to cut maintenance costs by 5%.
2. Digital twins
Machine learning technologies in manufacturing also play a great part in building digital twins. By using these so-called digital copies of a product, component, or process, a manufacturer can then conduct real-time diagnostics, find anomalies, and optimize processes.
Estimatedly, by 2027, over 40% of large companies will implement digital twins aiming at increased revenues. No wonder this technology is a trending use case of machine learning in manufacturing. Here are its most common applications:
- To simulate and validate a process, approach, or machine to find out how efficient it could be without spending company resources
- To build a digital twin of a production line to identify potential problems and bottlenecks and prepare for them in advance
- To test out various product designs before investing in a solid prototype
- To virtually monitor and manage performance on the factory floor
- To solve supply chain challenges, including route efficiency, packaging performance, and fleet management
- To improve knowledge transfer using more interactive applications in operator training
The world’s biggest and most acknowledged manufacturers get the advantage of digital twins, from Bosch to Siemens to Ford. Watch the video below to check how a digital supply chain twin from Bosch can help with process optimization and the removal of asymmetric information.
Another compelling example includes Unilever PLC, a consumer-good giant, that has built virtual versions of its factories to track and improve physical conditions such as temperature or motor speed.
3. Energy consumption forecasting
Manufacturing is among the major energy-consuming industries in the world, especially when it comes to electrical, food, metal, or plastic manufacturing. That’s why predicting energy consumption is one more critical touchpoint between manufacturing and machine learning.
The combined effort of IoT devices and machine learning allows manufacturers to gather enough historical data about lighting, humidity, activity levels of the facility, and so on, and then use these data to forecast and save up on energy consumption.
Read Also: IoT Data Analytics in Automotive Industry
Aside from reduced costs, a manufacturer can benefit from:
- Anomaly detection since high temperatures, abnormal humidity, etc. usually indicate potential equipment problems
- Energy reductions
- Long-term electricity demand projections
For example, E4SIGHT is an ML-based platform designed to automatically predict energy consumption for each connection point (electronic meter “POD”). Energy forecasts are based on real-time consumption data, as well as social media and satellite data.
4. Generative design
The concept of generative design means creating 3D design models and optimizing them with the help of AI. In this case, you as a manufacturer create as many design alternatives as needed, without wasting company resources. Then what’s left is to select the most suitable design option and put it into production.
Exploring other machine learning use cases in manufacturing, ML can be useful to complement generative design when a manufacturer has large datasets. If a manufacturer uses ML to create initial designs based on historical data, a company will likely get better and more relevant designs.
Generative design, accompanied by ML, is growing especially popular in the automotive industry. Here manufacturers try out various product designs to meet the needs of demanding consumers. Let’s check how Volkswagen Group incorporates generative design in its operations:
5. Predictive quality and yield
Predictive quality is one more application of machine learning in manufacturing. This way, a manufacturer discovers the hidden causes behind its production losses. By deriving insights from process data, the company can discover more about quality, yield, energy efficiency, waste, etc. Later these estimations help to make smarter, data-driven decisions.
The specific advantages of predictive quality in manufacturing include:
- More efficient production planning
- Less scrap is achieved by less rework and decreased use of materials
- Better quality assurance
- Improved process transparency
- Less waste due to fewer defective products
Check how to launch a predictive quality approach in manufacturing below.
The role of machine learning in manufacturing is increasing significantly, with more use cases appearing yearly. So the faster the company implements machine learning, the more opportunities it will get in the future.
Specifics of Developing ML models for Manufacturing
To get the most value from machine learning in manufacturing, a company should remember the next nuances:
- Get more quality data. ML models are data-driven, so the more quality data you get, the better your results will be. Industrial Internet of Things (IIoT) sensors are the most obvious data sources in the factory setting, but consider getting data from alternative sources too. For example, manufacturers can derive insights from PLC data (programmable logic controllers, i.e., industrial computers used for monitoring equipment), geospatial information, maintenance records, etc.
- Break down data siloes. In the manufacturing industry, siloed data is a common practice, which means there is limited access to industrial data. For instance, only one department or division may have access to particular datasets, and you as a manufacturer should consider options of how to use this “dark data” for your benefit.
- Ensure data transformations. Since you get your data from disparate sources, the data should be pre-processed and cleaned up to go into a machine learning model. In particular, team up with data scientists to remove all null, missing, or duplicate values as well as convert non-numeral values (like a string) into numerical ones to make them readable by ML algorithms. This is important to increase prediction accuracy further.
- Choose ML algorithms. Divide your data into two datasets — one for training the ML model and another for testing it. Your choice of ML algorithms will largely depend on what problems you’re going to solve. In manufacturing, this is usually either classification tasks (e.g. distinguishing between defective and non-defective parts) or regression ones (predicting when some equipment is going to degrade).
- Train the ML model. Feed the ML model with enough quality data, validate the results, and fine-tune the model if necessary. Once the ML model is ready, a manufacturer can wrap it up into the application so it was easier to use it.
- Maintain the ML solution. For better performance, a manufacturer should continue to maintain the solution properly and retrain the ML model if needed, for example, when new data appears.
- Teach the personnel. Remember that implementing the solution is only half the success. The other half depends on whether and how a manufacturer uses it. Make sure you pair up with ML engineers to teach your operators how to use the ML solution in the best way possible.
Benefits of ML in manufacturing
As you see, the adoption of AI and ML in business, in particular, can bring lots of value to your manufacturing business. Here are specific wins you can get:
-
Reduced cycle time and scrap and better resource utilization, for example, by predicting demand better and improving production schedules
-
Continuous quality improvement such as by using ML to find and remove defective products faster and avoid performance errors in machinery
-
Increased operational efficiency through tracking the entire manufacturing cycle, seeking, and removing bottlenecks
-
Reduced maintenance costs and improved reliability such as by extending life of equipment and machinery
-
Enhanced safety on the factory floor
Wrap up
Industry 4.0 is already here, bringing disrupting technologies into the manufacturing industry. In this article, we read about machine learning in manufacturing examples. As a subset of AI, machine learning enables businesses to reach unmatched performance, without compromising quality. So, isn’t it the best time to implement ML and revolutionize the way your business operates, and solve maintenance problems with machine learning techniques?
In case you need any assistance, our team of experienced developers is ready to support you throughout the whole AI/ML adoption journey.
If you’re interested in manufacturing software development or ML projects, it’s high time to get started. ML experts from Intelliarts AI’s team are here to help you. To learn more and overcome your competitors, feel free to reach out.
FAQ
1. How can machine learning help with defect detection in manufacturing?
Based on historical and real-time data, machine learning analyzes the equipment’s health state and seeks subtle differences in product characteristics that may then help in defect detection.
2. How can businesses get started with implementing machine learning in manufacturing?
A good starting point for businesses is to check your raw data and search for data gaps. Then, an efficient data collection process should be established since the quality and quantity of data are truly important when it comes to machine learning. Hiring a skillful ML team could be a good idea to avoid the typical mistakes, such as missing useful insights when looking at the data or not qualifying machine learning manufacturing use cases.
3. How quickly does the ML model start working in manufacturing?
To put an ML model into production takes at least a few months. Approximately after half a year, a manufacturer can expect the first results.
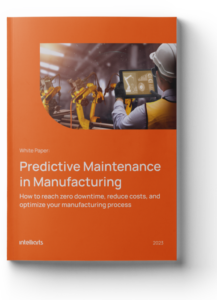