Probably, all businesses dealing with equipment assets struggle with their maintenance. Downtime, compromised performance, and high maintenance costs are some of the issues companies, including renewable energy assets providers, face. The solution is complementing a traditional maintenance system with predictive maintenance.
In this post, you’ll get an in-depth overview of the benefits and usage of predictive analytics in the renewable energy sector. Besides, you’ll explore a step-by-step implementation roadmap for predictive maintenance. It will help to form an understanding of how to get started with a predictive maintenance system for your renewable energy assets.
Overview of predictive maintenance in the renewable energy sector
Predictive maintenance in renewable energy is experiencing significant growth, driven by technology advancements like IoT and machine learning. — Oleksandr Stefanovskyi, a strategic technology partner at Intelliarts.
The global predictive maintenance market for the energy sector alone is projected to reach $1.79 billion in 2024 and is expected to grow at a compound annual growth rate (CAGR) of 25.77%, reaching $5.62 billion by 2029. As for the broader predictive maintenance market, it’s valued at $10.6 billion as of 2024 and is predicted to grow to as much as $47.8 billion by 2029.
As it’s possible to note, predictive maintenance, as an approach and technology, is of huge popularity across industries. Among other usages, it can be exploited with renewable energy equipment units of various types.
Examples of renewable energy assets that are frequently used in the industry and can benefit from predictive maintenance are provided in the image below:
Traditional vs. predictive maintenance method
Traditional maintenance is either a reactive or scheduled approach to maintaining equipment assets.
- Reactive maintenance: Fixing equipment immediately after it fails.
- Scheduled maintenance: Performing routine maintenance at fixed intervals regardless of asset condition.
While this method ensures some level of system upkeep, it can lead to unexpected downtime, unnecessary repairs, and higher operational costs, as issues are only addressed after a breakdown or on a schedule that may not reflect the actual condition of the equipment.
Predictive maintenance in the energy sector is a proactive approach that uses AI-driven analytics, real-time data from IoT sensors, and predictive models to anticipate when equipment is likely to fail.
predictive maintenance in renewable industry helps identify potential issues before they cause downtime or costly failures, unlike traditional maintenance. It optimizes the performance and longevity of equipment assets. This method improves efficiency, reduces unexpected maintenance costs, and increases operational reliability across the energy infrastructure.
You may explore predictive maintenance advantages and limitations in another blog post by the Intelliarts team.
Note: Predictive maintenance in the renewable industry is superior to traditional maintenance in several ways. However, it can’t replace regular maintenance methods. Predictive maintenance should be regarded as an enhancement and an addition to traditional maintenance rather than its complete replacement.
Benefits and use cases of predictive maintenance in renewable energy
Let’s proceed with reviewing the advantages and real-life applications of AI-driven predictive maintenance for renewable energy.
#1 Wind turbine monitoring
Predictive maintenance is used to monitor the condition of wind turbines, predicting mechanical failures before they happen. Sensors collect data on vibrations, temperature, and pressure, allowing operators to detect early signs of wear and prevent breakdowns.
Example: GE Renewable Energy implemented AI-driven predictive maintenance on its wind turbines, resulting in reduced downtime and enhanced operational efficiency.
#2 Solar panel performance optimization
Predictive analytics is used for remote monitoring and maintenance of solar panels, identifying factors like dirt accumulation, shading, or mechanical issues. This approach helps optimize energy production by scheduling necessary cleaning or repairs.
Example: First Solar uses predictive analytics in renewable energy to monitor solar farms, improving the longevity and efficiency of its panels.
#3 Hydroelectric power plants
In hydroelectric plants, AI-driven predictive maintenance for renewable energy prevents mechanical failures in turbines and generators by analyzing data from sensors that monitor key parameters like water flow and mechanical stress.
Example: Statkraft, a leading hydropower company, implements predictive maintenance to enhance efficiency and reduce unplanned downtime in its hydroelectric facilities.
#4 Biomass power plants
In biomass power plants, predictive maintenance systems monitor equipment like boilers and feed systems to detect signs of wear or malfunction before they cause shutdowns. This helps avoid unscheduled downtime and maintain energy production levels.
Example: Drax Group uses predictive maintenance for its biomass plants, improving reliability and reducing unexpected outages.
#5 Tidal and wave energy systems
Predictive maintenance is used in tidal and wave energy systems to monitor the harsh marine environment and detect potential failures in underwater components before they lead to costly repairs.
Example: Nova Innovation employs predictive maintenance in its tidal turbines to predict failures and optimize energy production in challenging marine environments.
You can explore the summarization of value-adding benefits that implementing predictive maintenance offers in the image below:
How to implement predictive maintenance in renewables — 6 steps
From Intelliarts experience, there are major steps to carry out before it even becomes possible to proceed with development and setting up a predictive maintenance software solution. Here’s a general implementation roadmap for predictive maintenance for renewable energy infrastructure:
Step 1: Initial assessment and data collection
Your team should get started with predictive maintenance implementation by conducting a thorough assessment of renewable energy assets. It allows us to understand current maintenance processes and identify key data points for predictive analysis. Collect historical data from wind turbines, solar panels, or other assets if you have the means to do so at this stage.
What to do:
- Identify critical equipment for predictive maintenance.
- Gather sensor data on equipment performance, weather conditions, and energy output.
- Analyze downtime and failure data.
Outcomes:
- Complete understanding of data sources.
- Clear overview of potential maintenance issues.
You may request high-tech consulting and infrastructure examination from an experienced software engineering agency to aid you at this step.
Step 2: Setting up monitoring infrastructure
Then, you should proceed with installing or upgrading existing sensors and IoT devices on renewable energy equipment, so they can collect real-time data reliably. Ensure the system supports remote monitoring and data storage for future analysis.
What to do:
- Deploy vibration sensors, temperature monitors, and energy output sensors.
- Integrate IoT systems with data platforms.
- Ensure continuous data flow to central servers.
You can explore IoT devices in renewable energy from the corresponding blog post written by Intelliarts experts.
Outcomes:
- Enhanced real-time data collection.
- Reliable system for monitoring equipment status.
Step 3: Data processing and integration
Once you have the means to receive monitoring data, you need to establish a data processing pipeline to clean, filter, and integrate collected data from sensors into a central database. Ensure compatibility between different sources of data.
What to do:
- Use software for data cleaning and transformation.
- Build a data integration system for real-time data handling.
- Enable API connections for third-party data sources.
Outcomes:
- Unified, clean dataset.
- Consistent data for predictive model input.
Step 4: Developing predictive models
Now you’ve reached the AI and ML development stage. Here, you need to build and train predictive models using historical and real-time data as training and testing datasets. The models should be specific to renewable energy asset types and conditions.
What to do:
- Conduct end-to-end ML development of a predictive model
- Leverage supervised learning with failure data to train models.
- Test models on different equipment types.
- Adjust models for environmental conditions like wind speed or sunlight.
Outcomes:
- A tailored predictive maintenance model for renewable energy scenarios.
- Accurate prediction of equipment failures.
Step 5: Pilot testing and validation
Run pilot tests of the predictive maintenance tools for renewable energy on a small scale before full deployment. Additionally, validate the accuracy of the predictive models by comparing predictions with actual performance data.
What to do:
- Select a few wind turbines, solar panels, or other equipment units for pilot testing.
- Continuously monitor model predictions vs. actual equipment performance.
- Adjust algorithms based on the results.
Outcomes:
- Proof of concept for predictive maintenance.
- Model fine-tuning based on real-world results.
Step 6: Full-scale deployment and optimization
In the final stage, you need to deploy the predictive maintenance system across all renewable energy assets, with continuous monitoring for system optimization. It’s essential to train your maintenance teams on how to interpret and act on predictive insights so they can translate ML-driven insights into actual predictive maintenance activities, like repair or part replacement.
What to do:
- Roll out the system across all wind farms or solar parks.
- Implement real-time dashboards for maintenance teams.
- Create a feedback loop for improving predictions based on outcomes.
- Educate the personnel on how to use the solution in their day-to-day work.
Outcomes:
- Fully operational predictive maintenance system.
- Reduced unplanned downtimes and increased efficiency.
You may also explore predictive maintenance in manufacturing from another blog post by Intelliarts.
Why Intelliarts for your predictive maintenance in renewable energy project
Intelliarts is an experienced team of AI and ML software engineers. We’ve been on the market for more than 24 years, offering tailored software solutions to various business needs. Known for a business-driven approach, we’ve built a solid reputation, achieving a 90% customer retention rate and fostering long-term partnerships — some lasting over 15 years.
Moreover, we have practical experience with predictive maintenance as well as with many forms of renewable energy solutions in business.
As such, in one of our projects we delivered an ML-powered comprehensive renewable energy solutions and maintenance services that can process data received from EV chargers, run anomaly analysis, and provide predictions regarding equipment condition and potential failure. Our solution provided our customer, EV Connect, with a notable maintenance process improvement, resulting in additional business value.
Explore predictive maintenance for EV chargers success story by Intelliarts.
In another of our case studies, we provided our customer with local predictive maintenance for renewable energy systems, which are basically power management solutions. They help schedule power limits for EV charging stations. This way, users can meet utility power constraints, minimize energy consumption, and extend charging infrastructure and charging equipment lifespan.
Explore the smart power management system development success story by Intelliarts.
The Intelliarts team is also known for our innovative projects in the renewable energy niche. Within the scope of our research and development initiatives, we created a computer vision-enabled ML model that can detect overheated spots in solar panels. You can give this computer vision model for solar panel anomaly detection a try yourself, as well as explore how it works in the video below:
Final take
As renewable energy demands grow, predictive maintenance driven by IoT and machine learning appears to be a suitable solution for optimizing performance and minimizing downtime across diverse energy assets. The implementation of predictive maintenance for renewable energy assets demands investment, effort, and expertise. Nevertheless, cost-efficiency and reliability it offers make predictive maintenance a promising initiative.
The Inteliarts team is ready, willing, and able to help you leverage the transformative power of predictive maintenance strategies for energy efficiency. With our vast experience and relevant expertise, we can advise on your project as well as provide quality software engineering services.
FAQ
1. What technologies and tools do you recommend for implementing predictive maintenance in our renewable energy systems?
For predictive maintenance in renewable energy, we recommend using AI-driven tools like IoT sensors for real-time data collection, machine learning algorithms to predict failures, and big data analytics platforms (e.g., AWS, Azure) to analyze performance. These technologies help monitor renewable energy infrastructure, improve asset reliability, and optimize maintenance schedules.
2. How do you ensure the data collected from our assets is secure and compliant with industry regulations?
We ensure data security and compliance for predictive maintenance in renewable energy systems through robust encryption, secure cloud platforms, and real-time monitoring. Our solutions also follow industry regulations like ISO 27001 for data security and GDPR for data privacy, ensuring your assets’ data is protected and meets regulatory standards.
3. How quickly can we expect to see a return on investment?
For AI-driven predictive maintenance for renewable energy systems, ROI can typically be observed within 6-12 months. By reducing downtime, minimizing maintenance costs, and extending asset lifespans, predictive analytics in renewable energy can significantly improve operational efficiency and offer cost savings in the long term.
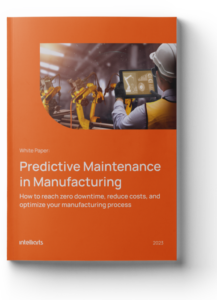